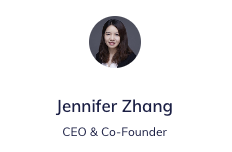
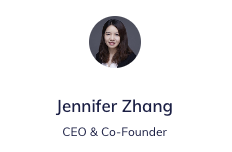
Recent advances in large language models (LLMs) are renovating the development of intelligent AI agents with powerful natural language understanding capabilities. This has sparked rising interest in LLM powered autonomous agent systems, or LLM agents.
According to Insignia Ventures Partners, the market for autonomous AI agents is estimated to grow at a CAGR of 43% (from USD 5 billion in 2023 to USD 29 billion by 2028), catalyzed by the democratization of LLMs. In this article, let’s explore what new possibilities LLM agents have unlocked and what are some feasible applications we can envision in the future.
*NOTE: For the simplicity of the article, we treat “LLM powered autonomous agent systems” and “LLM agents” as two interchangeable concepts. They belong to a bigger concept called AI agents. AI agents refer to Artificial Intelligence systems that can perceive environments and take autonomous actions to achieve goals.
What is LLM powered autonomous agent system
To answer the question, we can imagine LLM is the brain, and it can call different agents or tools, which are its hands and feet, to automatically perform a diverse range of tasks.
As Open AI’s Lilian Weng describes, a LLM powered autonomous agent system is comprised of an LLM functioning as the brain, and three other crucial components for planning, memory and tool use.
Planning
LLM agents can mimic human thinking patterns and proactively plan for task execution. During planning, LLM agents can break down large and complex tasks into smaller, manageable steps. They are also capable of self-reflecting and learning from past actions and mistakes, so as to optimize for future steps and improve final results.
Memory
This encompasses both short-term memory from in-context learning, as well as long-term memory from search and retrieval. Memory helps LLM agents to learn between context in real-time and recall information over extended timeframes.
Tool use
LLM agents can proactively call external APIs or vector stores for additional information, based on dynamic decision-making. By calling different tools and using semantic search and vector databases, LLMs agents can provide precise answers according to search results. This also avoids common LLM issues such as inaccuracy and hallucinations.
LLM agents: combining conversation capabilities with actions
LLMs have brought a new edge through their Natural Language Understanding (NLU) capabilities. This makes real human machine interaction a reality, and in natural languages! With LLMs, we can now communicate with machines just as we would with another human.
But LLMs alone can not realize a wide array of real-world applications. To unlock the full potential of LLMs, we need to build systems that can acquire and apply knowledge to solve practical problems. That’s where LLM powered autonomous agent system comes in. Without agents or tools, LLMs are like brains in vats-impressive but isolated from the real world.
Practical applications of LLM agents
Integrating LLMs into autonomous agent systems unlocks greater possibilities. Let’s look at two examples of LLM agents in action:
- Changing a client’s flight booking
Here the LLM agent must first understand what information is needed, like the airline’s change policy and available alternative flights (planning). It can then call tools like documentation APIs and flight databases to gather the necessary details (tool use).
- Transferring a hospital patient’s call
The LLM agent needs to comprehend what department the patient requested (short-term memory: in-context understanding). It can then check office hours, on-call numbers, and other relevant information (planning and tool use). This allows dynamically connecting the patient to the right destination, i.e. department extension during office hours, or the doctor on call during non-office hours.
The road ahead: from Software 2.0 to Enterprise Software 2.0
Intelligent LLM agents are taking a huge leap towards an era known as “Software 2.0”. This is a concept proposed by former Tesla director of AI, Andrej Karpathy. In Software 1.0, human engineers write codes and programs to complete tasks. These codes and programs are like individual dots with some desirable behavior.
While Software 2.0 refers to a new generation of software that leverages machine learning algorithms and neural networks to build intelligent, self-learning systems. Software 2.0 can analyze data, identify patterns, and constantly optimize its own code without human intervention.
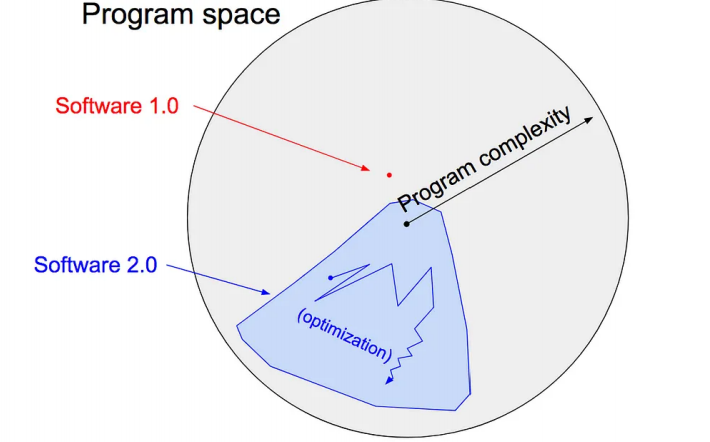
Applying the above concept into today’s enterprise software system, we see AI capabilities are currently serving as supporting tools to perform isolated tasks. These AI capabilities can include recommendation algorithms, Natural Language Processing (NLP), Text to Speech (TTS), Automatic Speech Recognition (ASR) and so on.
Looking ahead, we expect to enter an era we call “Enterprise Software 2.0”. In the era of Enterprise Software 2.0, LLM agents will become the core command center of the entire enterprise software system. In the system, LLMs are industry experts and decision makers. They would understand domain-specific enterprise knowledge and dynamically call different tools to automate task completions. The ecosystem is with close-loop communication and operation. The agents or tools being called by LLMs can vary, including common office systems such as CRM, ERP, OA and PMS etc.
By then, LLM agents will have the ability to assist in solving intricate issues across diverse industries, and self-learn from their own experience. We envision LLM agents to be versatile copilots, managing workflows alongside employees and assisting customers. AI accessibility will also be further democratized by LLM powered autonomous agent system, enabling anyone to copilot with a multitude of LLM agents to manage as many tasks as possible, and amplify productivity.
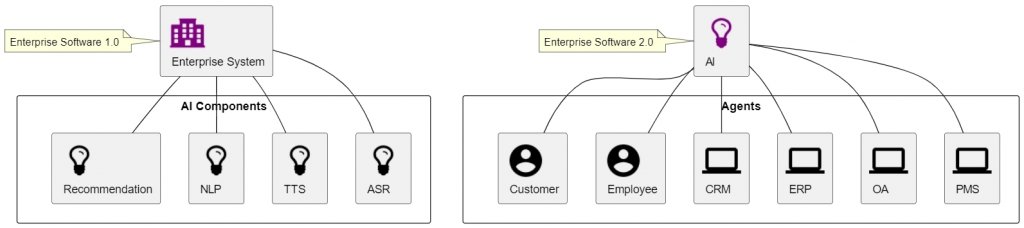
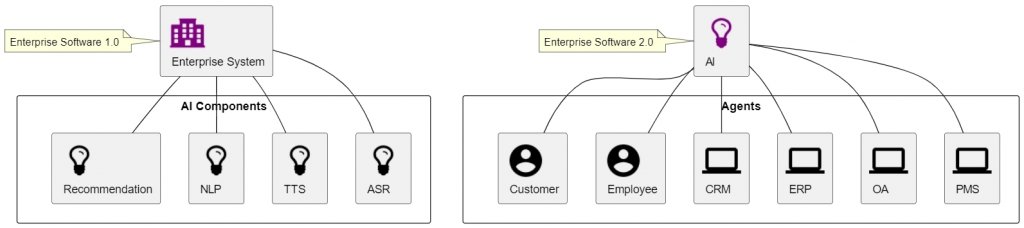
The road ahead remains long, but the disruptive potential is immense. LLM powered autonomous agent system has displayed transformative impact in dynamic, unstructured environments. Although limitations exist, steady progress in machine learning algorithms and design patterns will empower LLM agents to tackle ever more complex challenges. The future of AI has never looked more promising!
Reference
Lilian Weng. (2023). LLM Powered Autonomous Agents. Retrieved from: https://lilianweng.github.io/posts/2023-06-23-agent/
Wechat official accout. (2023). AI Agents大爆发:软件2.0雏形初现,OpenAI的下一步. (The rise of AI agents: first glimps of software 2.0 and what’s next for OpenAI). Retrieved from: https://mp.weixin.qq.com/s/Jb8HBbaKYXXxTSQOBsP5Wg
Andrej Karpathy. (2017). Software 2.0. Retrieved from: https://karpathy.medium.com/software-2-0-a64152b37c35